Every six seconds over many days, detectors have been recording the levels of different sizes of airborne particles near the main coal transport railway corridor through the Hunter Valley.
It’s part of a New South Wales Environment Protection Authority (EPA) study to gauge the impact of pollution from rail traffic on the health of residents—and a perfect application for a new way of creating models from enormous data sets, without losing the detail contained in the underlying data.
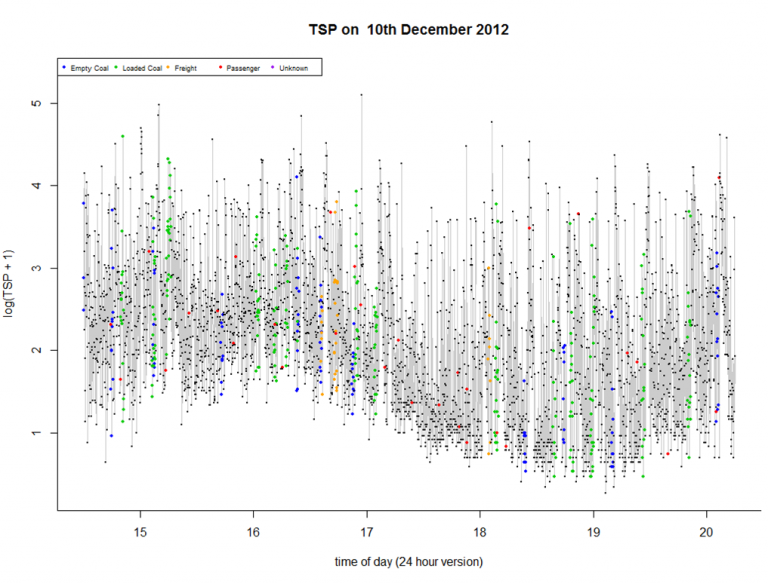
Coal-dust-particles
Snippet of large coal train dust exposure dataset, varying by train type and environmental variables, from the Hunter Valley region
As described in the ACEMS 2015 annual report, one investigation suggested that the pollution was largely due to dust thrown up by the vibrations of passing trains, no matter whether the trains were empty or full of cargo. Now the data is being explored further.
Collecting big data sets like this is the ‘easy’ part. Extracting useful information from it— how particulate pollution depends on the type and schedule of the trains, and what role the weather plays—is another story.

unsw_ci_scott_sisson.jpg
Prof Scott Sisson
“The theoretical paper mathematically validates what people have been doing in the past, but it also shows these methods have serious shortcomings. They don’t give results you’re actually interested in most of the time. In fact, people should be doing what we’re doing,” says Scott, who is from the School of Mathematics and Statistics at the University of New South Wales, and current President of the Statistical Society of Australia.
SCOTT SISSON NAMED AN AUSTRALIAN RESEARCH COUNCIL FUTURE FELLOW
Scott’s group works in a field known as symbolic data analysis. The classic way of dealing with problems of too much data is through summarising the measurements with symbols—mathematical representations that collapse the data and make it easier to handle. This approach is also used to deal with measurements presented in a nonstandard form, such as blood pressure which, because it changes constantly, is recorded not as a single figure, but in terms of maxima and minima over a short period of time.
Symbols ideally capture the essence of data in a simpler form and can be used to weed out meaningless and unnecessary data. They can then be processed using classical statistical techniques. At least that has been the theory.
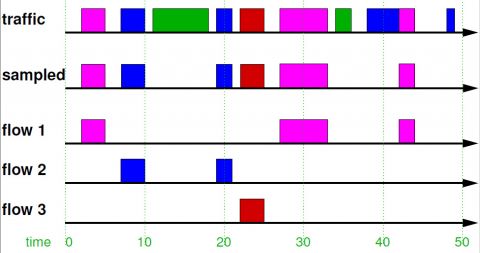
network-traffic-flow-sisson.jpg
Computer network traffic flow datasets, typically measured in petabytes/month, contain critical information on cyber security and network performance
In short, Scott’s group has developed ways of estimating how components of the underlying data mix and interact to produce the values of the symbols they observe. So the models they build relate more closely to what’s really happening.
The potential applications are enormous. In addition to the Hunter Valley data on which they are working in partnership with Chief Investigator Professor Louise Ryan from the University of Technology Sydney, Professor Matthew Roughan from the University of Adelaide (another ACEMS chief investigator) has provided Scott with the challenge of analysing data on computer network traffic to determine points of congestion and external attack. The group is also working with the Australian Institute of Marine Science—one of ACEMS’ partner organisations—on how best to estimate species abundances from diverse published data sources.