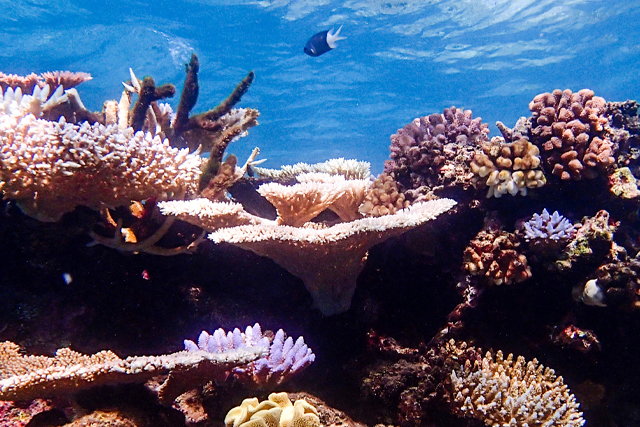
Case study: Numbers in the real world
Working with big data can be tricky. Environmental data can be trickier than most, as it often relies on irregular collections of sensors out in the wild, subject to weather and interactions with plants, animals and bacteria.
"The data are really complex," says Dr Erin Peterson at QUT, an environmental scientist who developed an interest in statistics when she realised that existing techniques couldn't describe the river systems she was studying.
"It might be collected at different time intervals and at different spatial scales. You have mismatches, you have a lot of anomalies. The uncertainties associated with the different types of measurements are all different, the methods of collection are all different."
Erin was doing her PhD, on environmental monitoring for rivers, when she first encountered statistical challenges.
All the methods for studying spatial patterns in the environment were developed for Euclidean space. But rivers branch, like trees.
When Erin realised that the methods she needed didn't exist, she teamed up with statisticians to develop tools for modelling data from river networks.
Erin's current work still involves some of the same questions: given a limited amount of data of varied quality, how can you develop the most accurate picture of the real world?
One of Erin's recent projects, developed in collaboration with Professor Kerrie Mengersen and others, is Virtual Reef Diver. Chosen as the ABC Science citizen science project in 2018, Virtual Reef Diver invites members of the public to help monitor the health of the Great Barrier Reef.
"People register to look at underwater images and we ask them to describe what they see at certain points. We use that to get an estimate of coral cover in the image and then we can estimate reef health."
The responses are automatically processed and combined with data from professional monitoring organisations.
"There are more than 65 organisations out there monitoring the Reef. Many collect images but they all do it in different ways and classify it in different ways."
All the data are brought together into a continuously updated statistical model that gives estimates of the coral cover across the entire Reef, even where there are no data. Crucially, it also includes information about uncertainty in the estimates at each point.
"Anyone can download the results. You can imagine organisations might start to focus their sampling in areas where there is little data or high levels of uncertainty. You can also see how the coral cover changes through time, which could be useful to identify things like outbreaks of crown of thorns starfish."
The project has so far been a success, with more than 100,000 images classified last August alone. A second phase, which allows people to upload their own photos, launched recently.
Another project, in collaboration with the Queensland Department of Environment and Science and Monash University, is about monitoring water quality in rivers that flow out onto the Reef.
The end goal is to develop an automated sensor network that delivers data in real time about sediment and nutrient levels in the water across the river system.
One obstacle is cost. A single sensor to detect nitrates – a common nutrient carried in fertiliser runoff, which can feed algal blooms or crown of thorns starfish outbreaks – can cost as much as $20,000.
To bring costs down, Erin and others have devised ways to use data from temperature and conductivity sensors to produce estimates of sediment and nutrient concentrations in water.
Reliability is another issue.
"You get anomalies, like batteries running out or algae growing on the sensors."
Working with several anomaly detection algorithms, including some developed by Monash PhD student Dilini Talagala, Erin and colleagues have developed a quick automated system for figuring out when a sensor's data may be untrustworthy. The next step is to move toward applying anomaly detection algorithms to sensors deployed across the whole catchment.